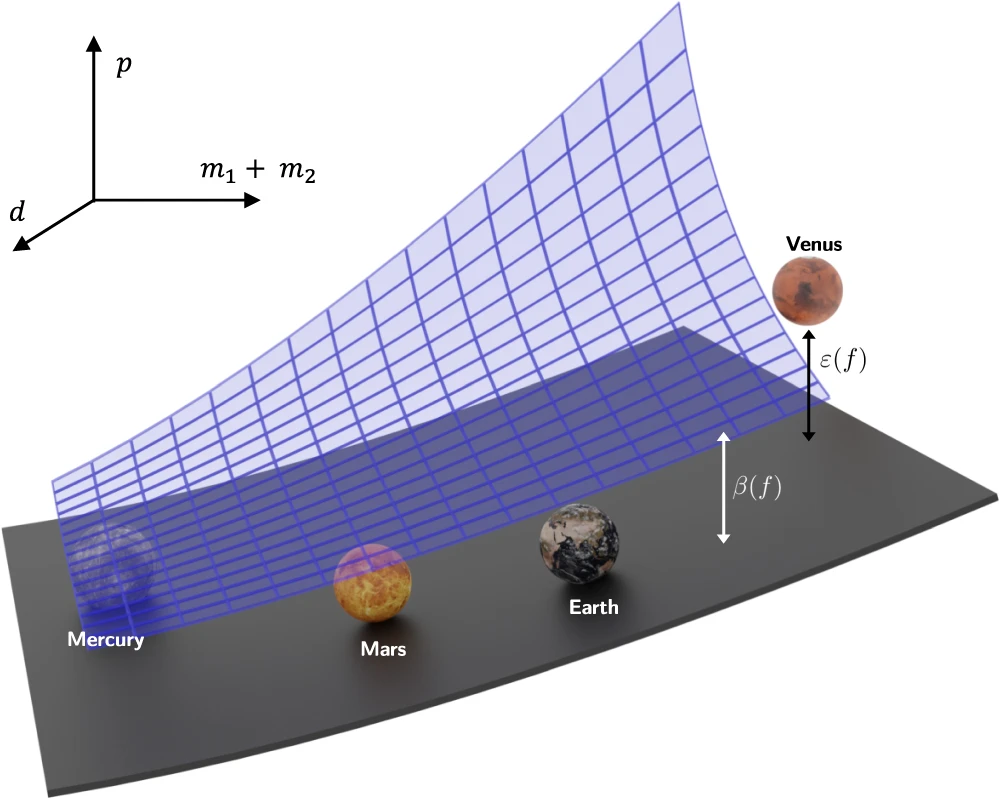
In 1918, the American chemist Irving Langmuir published a paper examining the behavior of gas molecules sticking to a solid surface. Guided by the results of careful experiments, as well as his theory that solids offer discrete sites for the gas molecules to fill, he worked out a series of equations that describe how much gas will stick, given the pressure.
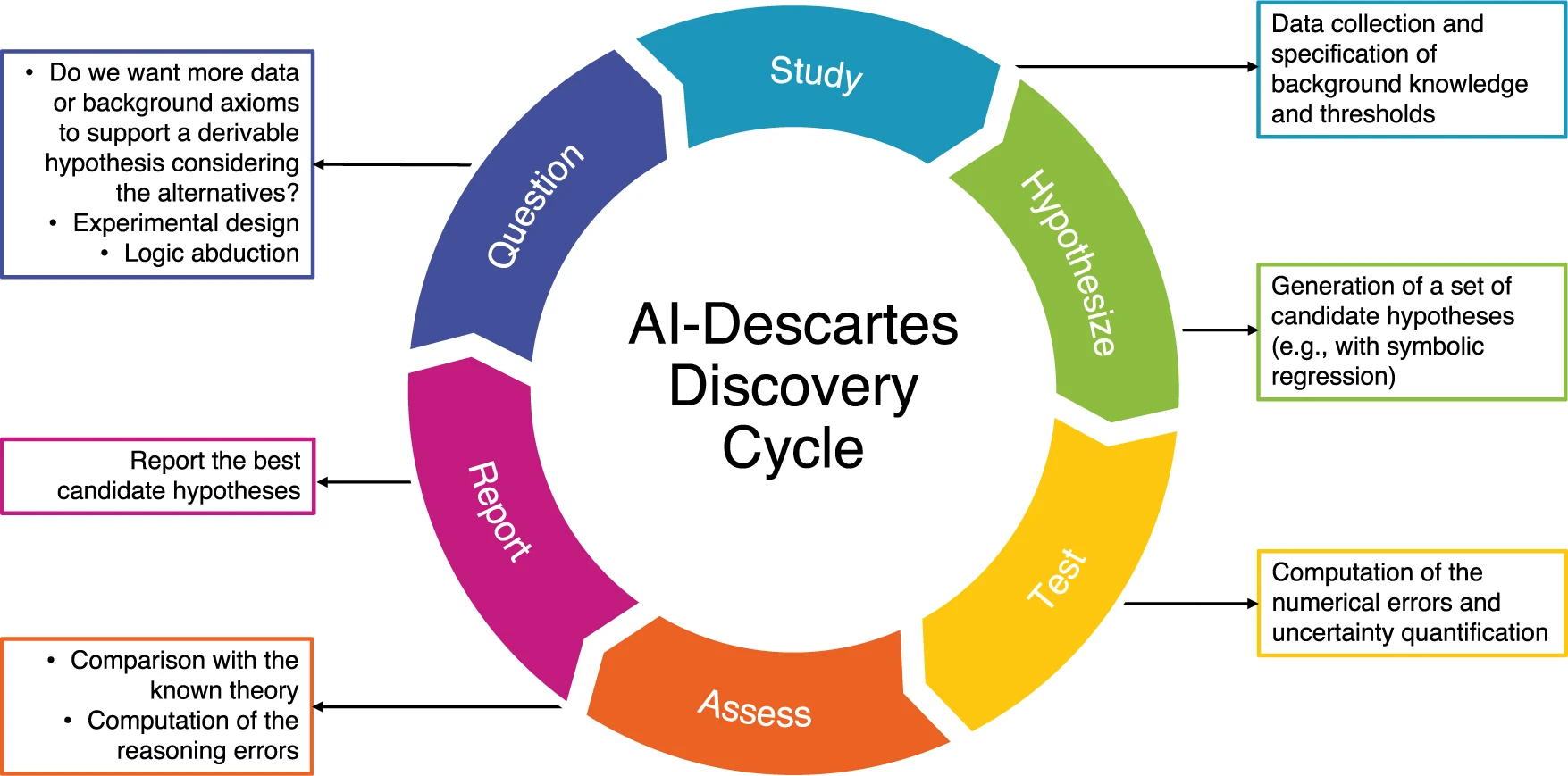
Now, about a hundred years later, an “AI scientist” developed by researchers at IBM Research, Samsung AI, and the University of Maryland, Baltimore County (UMBC) has reproduced a key part of Langmuir’s Nobel Prize-winning work. The system—artificial intelligence (AI) functioning as a scientist—also rediscovered Kepler’s third law of planetary motion, which can calculate the time it takes one space object to orbit another given the distance separating them, and produced a good approximation of Einstein’s relativistic time-dilation law, which shows that time slows down for fast-moving objects.
The research was supported by the Defense Advanced Research Projects Agency (DARPA). A paper describing the results will be published in the journal Nature Communications on April 12.
A machine-learning tool that reasons
The new AI scientist—dubbed “AI-Descartes” by the researchers—joins the likes of AI Feynman and other recently developed computing tools that aim to speed up scientific discovery. At the core of these systems is a concept called symbolic regression, which finds equations to fit data. Given basic operators, such as addition, multiplication, and division, the systems can generate hundreds to millions of candidate equations, searching for the ones that most accurately describe the relationships in the data.
AI-Descartes offers a few advantages over other systems, but its most distinctive feature is its ability to logically reason, says Cristina Cornelio, a research scientist at Samsung AI in Cambridge, England who is first author on the paper. If there are multiple candidate equations that fit the data well, the system identifies which equations fit best with background scientific theory. The ability to reason also distinguishes the system from “generative AI” programs such as ChatGPT, whose large language model has limited logical skills and sometimes messes up basic math.
“In our work, we are merging a first-principles approach, which has been used by scientists for centuries to derive new formulas from existing background theories, with a data-driven approach that is more common in the machine learning era,” Cornelio says. “This combination allows us to take advantage of both approaches and create more accurate and meaningful models for a wide range of applications.”
The name AI-Descartes is a nod to 17th-century mathematician and philosopher René Descartes, who argued that the natural world could be described by a few fundamental physical laws and that logical deduction played a key role in scientific discovery.
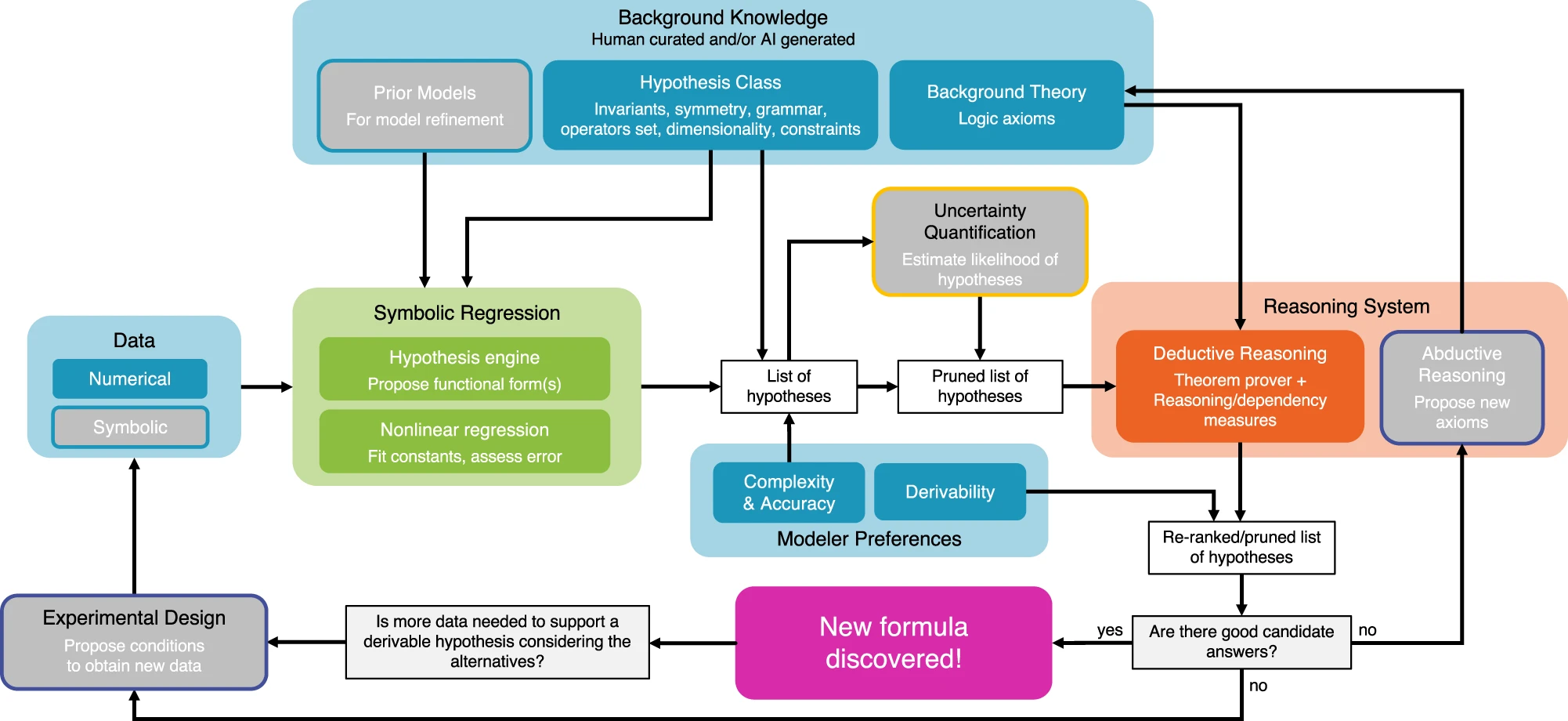
Suited for real-world data
The system works particularly well on noisy, real-world data, which can trip up traditional symbolic regression programs that might overlook the real signal in an effort to find formulas that capture every errant zig and zag of the data. It also handles small data sets well, even finding reliable equations when fed as few as ten data points.
One factor that might slow down the adoption of a tool like AI-Descartes for frontier science is the need to identify and code associated background theory for open scientific questions. The team is working to create new datasets that contain both real measurement data and an associated background theory to refine their system and test it on new terrain.
They would also like to eventually train computers to read scientific papers and construct the background theory themselves.
“In this work, we needed human experts to write down, in formal, computer-readable terms, what the axioms of the background theory are, and if the human missed any or got any of those wrong, the system won’t work,” says co-author Tyler Josephson, assistant professor of Chemical, Biochemical and Environmental Engineering at UMBC. “In the future,” he says, “we’d like to automate this part of the work as well, so we can explore many more areas of science and engineering.”
This goal motivates Josephson’s research on AI tools to advance chemical engineering.
Ultimately, the team hopes their AI-Descartes, like the real person, may inspire a productive new approach to science. “One of the most exciting aspects of our work is the potential to make significant advances in scientific research,” Cornelio says.